Saturday, December 22, 2007
Cheer, another accepted!
Sunday, November 18, 2007
GroupLens: A group good at Recommendation
Thursday, November 08, 2007
21st Canadian AI (2008) CFP
Friday, September 28, 2007
Begin to learn Lucene
Wednesday, September 19, 2007
Tuesday, September 18, 2007
SUMM: Something to compete with Conjoint Analysis
- SUMM can handle three or four times as many attributes as Conjoint because of its unique measurement scale.
- SUMM incorporates each respondent's subjective beliefs about vendors in each choice simulation, while Conjoint requires each product to be defined objectively.
- SUMM flows from a straightforward theory of how people make choices, so it is easier to understand how the numbers are generated.
You can find more about SUMM on Eric Marder Associates.
Monday, September 17, 2007
Powerful Conjoint Analysis
Monday, September 10, 2007
1st Educational Data Mining (EDM) (2008)
First International Conference on Educational Data Mining
Data Mining and Statistics in Service of Education
Call for papers (preliminary)
http://www.EducationalDataMining.org
Co-located with International Conference on Intelligent Tutoring Systems (ITS 2008)
The First International Conference on Educational Data Mining brings together researchers from computer science, education, psychology, psychometrics, and statistics to analyze large data sets to answer educational research questions. The increase in instrumented educational software, as well as state databases of student test scores, has created large repositories of data reflecting how students learn. The EDM conference focuses on computational approaches for using those data to address important educational questions. The broad collection of research disciplines ensures cross fertilization of ideas, with the central questions of educational research serving as a unifying focus. This Conference emerges from preceding EDM workshops at the AAAI, AIED, ICALT, ITS, and UM conferences.
Topics of Interest
We welcome papers describing original work. Areas of interest include but are not limited to:
· Improving educational software. Many large educational data sets are generated by computer software. Can we use our discoveries to improve the software’s effectiveness?
· Domain representation. How do learners represent the domain? Does this representation shift as a result of instruction? Do different subpopulations represent the domain differently?
· Evaluating teaching interventions. Student learning data provides a powerful mechanism for determining which teaching actions are successful. How can we best use such data?
· Emotion, affect, and choice. The student’s level of interest and willingness to be a partner in the educational process is critical. Can we detect when students are bored and uninterested? What other affective states or student choices should we track?
· Integrating data mining and pedagogical theory. Data mining typically involves searching a large space of models. Can we use existing educational and psychological knowledge to better focus our search?
· Improving teacher support. What types of assessment information would help teachers? What types of instructional suggestions are both feasible to generate and would be welcomed by teachers?
· Replication studies. We are especially interested in papers that apply a previously used technique to a new domain, or that reanalyze an existing data set with a new technique.
Important Dates (tentative)
· Paper submissions:
· Acceptance notification:
· Camera ready paper:
· Conference:
Submission types:
· Full papers: Maximum of 10 pages. Should describe substantial, unpublished work.
Sunday, September 09, 2007
Promote one site on the research on Conjoint Analysis
Welcome to post more good resource here.
Thanks!
Spatial Database and Data Mining
Wednesday, September 05, 2007
Normalized Google Distance (NGD)
Sunday, September 02, 2007
Paper accepted by Australian AI 2007
Tuesday, August 28, 2007
What is Hierarchical Bayes
Thursday, August 23, 2007
Standards to evaluate Conjoint Analysis
2. High accuracy in prediction to the survey initiator.
Sunday, August 19, 2007
CFP: FLAIRS-21(2008)
Thursday, August 16, 2007
Two new terms met recently
Tuesday, July 31, 2007
D-separation Tutorial
Wednesday, July 25, 2007
Computational Intelligence
One research center about CI: http://www.cs.vu.nl/ci/
Thursday, July 19, 2007
Markov Equivalent Class Graph
1. Same link and
2. Same uncoupled head-to-head meetings
Any assignment of directions to the undirected edges in this graph, that does not create a new uncoupled head-to-head meeting or a directed cycle, yields a member of the equivalence class.
(Find detail on http://mmc36.informatik.uni-augsburg.de/mediawiki/data/3/3a/SS07_MM2_Lec06-Bayesian_Networks_chapter02_Part2.pdf )
Monday, July 16, 2007
Sunday, June 10, 2007
CFP: AIKED'08
Acceptance notification: December 31, 2007
Conference date: February 20-22, 2008
Location: Cambridge University
AIKED'08 = The 7th WSEAS International Conference on Artificial Intelligence, Knowledge Engineering and Data bases
Good tutorial on classification tree
It covers primary steps involved in known versions of classification tree.
Enjoy it!
Tutorial of Artificial Neural Network
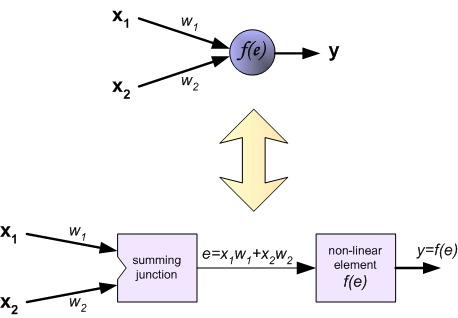
Enjoy it.
Saturday, June 09, 2007
Australian AI 2007
Notification date: August 31st, 2007
Conference date: December 2nd-6th, 2007
Location: Queensland's Gold Coast
Ranking of AI and related conferences
- AAAI: American Association for AI National Conference
- CVPR: IEEE Conf on Comp Vision and Pattern Recognition
- IJCAI: Intl Joint Conf on AIICCV: Intl Conf on Computer Vision
- ICML: Intl Conf on Machine Learning
- KDD: Knowledge Discovery and Data Mining
- KR: Intl Conf on Principles of KR & Reasoning
- NIPS: Neural Information Processing Systems
- UAI: Conference on Uncertainty in AI
- ICAA: International Conference on Autonomous Agents
- ACL: Annual Meeting of the ACL (Association of Computational Linguistics)
Rank 2:
- NAACL: North American Chapter of the ACL
- AID: Intl Conf on AI in Design
- AI-ED: World Conference on AI in Education
- CAIP: Inttl Conf on Comp. Analysis of Images and Patterns
- CSSAC: Cognitive Science Society Annual Conference
- ECCV: European Conference on Computer Vision
- EAI: European Conf on AI
- EML: European Conf on Machine Learning
- GP: Genetic Programming Conference
- IAAI: Innovative Applications in AI
- ICIP: Intl Conf on Image Processing
- ICNN/IJCNN: Intl (Joint) Conference on Neural Networks
- ICPR: Intl Conf on Pattern Recognition
- ICDAR: International Conference on Document Analysis and - Recognition
- ICTAI: IEEE conference on Tools with AI
- AMAI: Artificial Intelligence and Maths
- DAS: International Workshop on Document Analysis Systems
- WACV: IEEE Workshop on Apps of Computer Vision
- COLING: International Conference on Computational Liguistics
- EMNLP: Empirical Methods in Natural Language Processing
- EACL: Annual Meeting of European Association Computational - Lingustics
- CoNLL: Conference on Natural Language Learning
Rank 3:
- PRICAI: Pacific Rim Intl Conf on AI
- AAI: Australian National Conf on AI
- ACCV: Asian Conference on Computer Vision
- AI*IA: Congress of the Italian Assoc for AI
- ANNIE: Artificial Neural Networks in Engineering
- ANZIIS: Australian/NZ Conf on Intelligent Inf. Systems
- CAIA: Conf on AI for Applications
- CAAI: Canadian Artificial Intelligence Conference
- ASADM: Chicago ASA Data Mining Conf: A Hard Look at DM
- EPIA: Portuguese Conference on Artificial Intelligence
- FCKAML: French Conf on Know. Acquisition & Machine Learning
- ICANN: International Conf on Artificial Neural Networks
- ICCB: International Conference on Case-Based Reasoning
- ICGA: International Conference on Genetic Algorithms
- ICONIP: Intl Conf on Neural Information Processing
- IEA/AIE: Intl Conf on Ind. & Eng. Apps of AI & Expert Sys
- ICMS: International Conference on Multiagent Systems
- ICPS: International conference on Planning Systems
- IWANN: Intl Work-Conf on Art & Natural Neural Networks
- PACES: Pacific Asian Conference on Expert Systems
- SCAI: Scandinavian Conference on Artifical Intelligence
- SPICIS: Singapore Intl Conf on Intelligent System
- PAKDD: Pacific-Asia Conf on Know. Discovery & Data Mining
- SMC: IEEE Intl Conf on Systems, Man and Cybernetics
- PAKDDM: Practical App of Knowledge Discovery & Data Mining
- WCNN: The World Congress on Neural Networks
- WCES: World Congress on Expert Systems
- INBS: IEEE Intl Symp on Intell. in Neural \& Bio Systems
- ASC: Intl Conf on AI and Soft Computing
- PACLIC: Pacific Asia Conference on Language, Information and Computation
- ICCC: International Conference on Chinese Computing
- ICADL: International Conference on Asian Digital Libraries
- RANLP: Recent Advances in Natural Language Processing
- NLPRS: Natural Language Pacific Rim Symposium
PRICAI 2008
Submission due date: May 26, 2008
Notification date: July 25, 2008
Conference data: Dec. 15-19, 2008
Location: Hanoi, Vietnam
IEA/AIE 2008
Submission due date: Nov.8, 2007
Notification date: Feb. 1, 2008
Conference date: June 18-20, 2008
Location: Wroclaw, Poland
Wednesday, June 06, 2007
Boosting
From this URL http://www.cs.princeton.edu/~schapire/boost.html, you can find several introduction articles about boosting. It is a ongoing project in U. of Princeton.
Tuesday, June 05, 2007
Jobs for phd and post-doc.
PAKDD 2008
Due date: Sep. 30, 2007
Notification: Dec. 27, 2007
Conference date: May 20-23, 2008
Location: Osaka, Japan
http://www.ar.sanken.osaka-u.ac.jp/pakdd2008/cf_dates.html
Self-learning Respnose Model
We are working to provide better solutions for our users distributed all over the world.
3rd WSEAS International Symposimum on Data Mining and Intelligencet Information Processing
Notification of acceptance: July 30, 2007
Location: Beijing, P.R.China
Sunday, May 06, 2007
PAMI center - University of Waterloo
Saturday, April 28, 2007
Java Data Mining
Friday, April 27, 2007
PMML
Training provided to new pies in company
Wish them can take full responsibility asap!
Poster presentation on Canadian AI 2007
IAMB is really really data inefficient
I am still working to find a better solution, and seems PCMB is not bad one. But PCMB is too careful in the checking, which costs us much time in computing.
Bayes' Theorem
Bayes's Theorem is a simple mathematical formula used for calculating conditional probabilities. It figures prominently in subjectivist or Bayesian approaches to epistemology, statistics, and inductive logic. Subjectivists, who maintain that rational belief is governed by the laws of probability, lean heavily on conditional probabilities in their theories of evidence and their models of empirical learning. Bayes's Theorem is central to these enterprises both because it simplifies the calculation of conditional probabilities and because it clarifies significant features of subjectivist position. Indeed, the Theorem's central insight — that a hypothesis is confirmed by any body data that its truth renders probable — is the cornerstone of all subjectivist methodology.
1. Conditional Probabilities and Bayes's Theorem
2. Special Forms of Bayes's Theorem
3. The Role of Bayes's Theorem in Subjectivist Accounts of Evidence
4. The Role of Bayes's Theorem in Subjectivist Models of Learning
Bibliography
Other Internet Resources
Related Entries
You can read the whole text on http://plato.stanford.edu/entries/bayes-theorem/
Enjoy it.
Wednesday, January 10, 2007
Bayesian Networks? Who care that?
The future of software may lie in the obscure theories of an 18th century cleric named Thomas Bayes.
By LESLIE HELM, Times Staff Writer
When Microsoft Senior Vice President Steve Ballmer first heard his company was planning to make a huge investment in an Internet service offering movie reviews and local entertainment information in major cities across the nation, he went to Chairman Bill Gates with his concerns.
After all, Ballmer has billions of dollars of his own money in Microsoft stock, and entertainment isn't exactly the company's strong point.
But Gates dismissed such reservations. Microsoft's competitive advantage, he responded, was its expertise in "Bayesian networks." Asked recently when computers would finally begin to understand human speech, Gates began discussing the critical role of "Bayesian" systems. Ask any other software executive about anything "Bayesian" and you're liable to get a blank stare.
Is Gates onto something? Is this alien-sounding technology Microsoft's new secret weapon?
Quite possibly.
Bayesian networks are complex diagrams that organize the body of knowledge in any given area by mapping out cause-and-effect relationships among key variables and encoding them with numbers that represent the extent to which one variable is likely to affect another.
Programmed into computers, these systems can automatically generate optimal predictions or decisions even when key pieces of information are missing.
When Microsoft in 1993 hired Eric Horvitz, David Heckerman and Jack Breese, pioneers in the development of Bayesian systems, colleagues in the field were surprised. The field was still an obscure, largely academic enterprise. Today the field is still obscure. But scratch the surface of a range of new Microsoft products and you're likely to find Bayesian networks embedded in the software. And Bayesian nets are being built into models that are used to predict oil and stock prices, control the space shuttle and diagnose disease.
Artificial intelligence (AI) experts, who saw their field discredited in the early 1980s after promising a wave of "thinking" computers that they ultimately couldn't produce, believe widening acceptance of the Bayesian approach could herald a renaissance in the field.
Bayesian networks provide "an overarching graphical framework" that brings together diverse elements of AI and increases the range of its likely application to the real world, says Michael Jordon, professor of brain and cognitive science at the Massachusetts Institute of Technology.
Microsoft is unquestionably the most aggressive in exploiting the new approach. The company offers a free Web service that helps customers diagnose printing problems with their computers and recommends the quickest way to resolve them. Another Web service helps parents diagnose their children's health problems.
The latest version of Microsoft Office software uses the technology to offer a user help based on past experience, how the mouse is being moved and what task is being done. "If his actions show he is distracted, he is likely to need help," Horvitz says. "If he's been working on a chart, chances are he needs help formatting the chart." "Gates likes to talk about how computers are now deaf, dumb, blind and clueless. The Bayesian stuff helps deal with the clueless part," says Daniel T. Ling, director of Microsoft's research division and a former IBM scientist.
Bayesian networks get their name from the Rev. Thomas Bayes, who wrote an essay, posthumously published in 1763, that offered a mathematical formula for calculating probabilities among several variables that are causally related but for which--unlike calculating the probability of a coin landing on heads or tails--the relationships can't easily be derived by experimentation.
Early students of probability applied the ideas to discussions about the existence of God or efforts to improve their odds in gambling. Much later, social scientists used it to help clarify the key factors influencing a particular event. But it was the rapid progress in computer power and the development of key mathematical equations that made it possible for the first time, in the late 1980s, to compute Bayesian networks with enough variables that they were useful in practical applications.
The Bayesian approach filled a void in the decades-long effort to add intelligence to computers.
In the late 1970s and '80s, reacting to the "brute force" approach to problem solving by early users of computers, proponents of the emerging field of artificial intelligence began developing software programs using rule-based, if-then propositions. But the systems took time to put together and didn't work well if, as was frequently the case, you couldn't answer all the computer's questions clearly.
Later companies began using a technique called "neural nets" in which a computer would be presented with huge amounts of data on a particular problem and programmed to pull out patterns. A computer fed with a big stack of X-rays and told whether or not cancer was present in each case would pick out patterns that would then be used to interpret X-rays. But the neural nets won't help predict the unforeseen. You can't train a neural net to identify an incoming missile or plane because you could never get sufficient data to train the system. In part because of these limitations, a slew of companies that popped up in the early 1980s to sell artificial intelligence systems virtually all went bankrupt.
Many AI techniques continued to be used. Credit card companies, for example, began routinely using neural networks to pick out transactions that don't look right based on a consumer's past behavior. But increasingly, AI was regarded as a tool with limited use. Then, in the late 1980s--spurred by the early work of Judea Pearl, a professor of computer science at UCLA, and breakthrough mathematical equations by Danish researchers--AI researchers discovered that Bayesian networks offered an efficient way to deal with the lack or ambiguity of information that has hampered previous systems.
Horvitz and his two Microsoft colleagues, who were then classmates at Stanford University, began building Bayesian networks to help diagnose the condition of patients without turning to surgery. The approach was efficient, says Horvitz, because you could combine historical data, which had been meticulously gathered, with the less precise but more intuitive knowledge of experts on how things work to get the optimal answer given the information available at a given time. Horvitz, who with two colleagues founded Knowledge Industries to develop tools for developing Bayesian networks, says he and the others left the company to join Microsoft in part because they wanted to see their theoretical work more broadly applied. Although the company did important work for the National Aeronautics and Space Administration and on medical diagnostics, Horvitz says, "It's not like your grandmother will use it."
Microsoft's activities in the field are now helping to build a groundswell of support for Bayesian ideas. "People look up to Microsoft," says Pearl, who wrote one of the key early texts on Bayesian networks in 1988 and has become an unofficial spokesman for the field. "They've given a boost to the whole area." A researcher at German conglomerate Siemens says Microsoft's work has drawn the attention of his superiors, who are now looking seriously at applying Bayesian concepts to a range of industrial applications.
Scott Musman, a computer consultant in Arlington, Va., recently designed a Bayesian network for the Navy that can identify enemy missiles, aircraft or vessels and recommend which weapons could be used most advantageously against incoming targets. Musman says previous attempts using traditional mathematical approaches on state-of-the-art computers would get the right answer but would take two to three minutes. "But you only have 30 seconds before the missile has hit you," says Musman.
General Electric is using Bayesian techniques to develop a system that will take information from sensors attached to an engine and, based on expert opinion built into the system as well as vast amounts of data on past engine performance, pinpoint emerging problems. Microsoft is working on techniques that will enable the Bayesian networks to "learn" or update themselves automatically based on new knowledge, a task that is currently cumbersome. The company is also working on using Bayesian techniques to improve upon popular AI approaches such as "data mining" and "collaborative filtering" that help draw out relevant pieces of information from massive databases. The latter will be used by Microsoft in its new online entertainment service to help people identify the kind of restaurants or entertainment they are most likely to enjoy. Still, as effective as they are proving to be in early use, Bayesian networks face an uphill battle in gaining broad acceptance. "An effective solution came just as the bloom had come off the AI rose," says Peter Hart, head of Ricoh's California Research Center at Menlo Park, a pioneer of AI. And skeptics insist any computer reasoning system will always fall short of people's expectations because of the computer's tendency to miss what is often obvious to the human expert.
Still, Hart believes the technology will catch on because it is cost-effective. Hart developed a Bayesian-based system that enabled Ricoh's copier help desk to answer twice the number of customer questions in almost half the time. Hart says Ricoh is now looking at embedding the networks in products so customers can see for themselves what the likely problems are. He believes auto makers will soon build Bayesian nets into cars that predict when various components of a car need to be repaired or replaced.
Friday, January 05, 2007
How to generate X of geometric random variable
Another way to generate a random permutation
One method to generate a random permutation
Step2. Set k = n;
Step3. Generate a random number U and let I=Int(kU)+1;
Step4. Interchange the values of P1 and Pk;
Step5. Let k = k-1 and if k>1, go to Step 3;
Step6. P1, ..., Pn is the desired random permutation.
Here U is generated from uniform distribution. Advantage of this method include:
1) No extra memory cost since all operations are done on the original array. Only switch happens;
2) Algorithm complexity is determined by the length of permutation;
3) It is indeed random;
However, effective algorithm to get U is important here.
Monday, January 01, 2007
Social Network Analysis: A Brief Introduction
To understand networks and their participants, we evaluate the location of actors in the network. Measuring the network location is finding the centrality of a node. These measures give us insight into the various roles and groupings in a network -- who are the connectors, mavens, leaders, bridges, isolates, where are the clusters and who is in them, who is in the core of the network, and who is on the periphery?
We look at a social network -- the "Kite Network" above -- developed by David Krackhardt, a leading researcher in social networks. Two nodes are connected if they regularly talk to each other, or interact in some way. Andre regularly interacts with Carol, but not with Ike. Therefore Andre and Carol are connected, but there is no link drawn between Andre and Ike. This network effectively shows the distinction between the three most popular individual centrality measures: Degree Centrality, Betweenness Centrality, and Closeness Centrality.
Degree Centrality
Social network researchers measure network activity for a node by using the concept of degrees -- the number of direct connections a node has. In the kite network above, Diane has the most direct connections in the network, making hers the most active node in the network. She is a 'connector' or 'hub' in this network. Common wisdom in personal networks is "the more connections, the better." This is not always so. What really matters is where those connections lead to -- and how they connect the otherwise unconnected! Here Diane has connections only to others in her immediate cluster -- her clique. She connects only those who are already connected to each other.
Betweenness Centrality
While Diane has many direct ties, Heather has few direct connections -- fewer than the average in the network. Yet, in may ways, she has one of the best locations in the network -- she is between two important constituencies. She plays a 'broker' role in the network. The good news is that she plays a powerful role in the network, the bad news is that she is a single point of failure. Without her, Ike and Jane would be cut off from information and knowledge in Diane's cluster. A node with high betweenness has great influence over what flows in the network. As in Real Estate, the "golden rule" of networks is: Location, Location, Location.
Closeness Centrality
Fernando and Garth have fewer connections than Diane, yet the pattern of their direct and indirect ties allow them to access all the nodes in the network more quickly than anyone else. They have the shortest paths to all others -- they are close to everyone else. They are in an excellent position to monitor the information flow in the network -- they have the best visibility into what is happening in the network.
Network Centralization
Individual network centralities provide insight into the individual's location in the network. The relationship between the centralities of all nodes can reveal much about the overall network structure.
A very centralized network is dominated by one or a few very central nodes. If these nodes are removed or damaged, the network quickly fragments into unconnected sub-networks. A highly central node can become a single point of failure. A network centralized around a well connected hub can fail abruptly if that hub is disabled or removed. Hubs are nodes with high degree and betweeness centrality.
A less centralized network has no single points of failure. It is resilient in the face of many intentional attacks or random failures -- many nodes or links can fail while allowing the remaining nodes to still reach each other over other network paths. Networks of low centralization fail gracefully.
Network Reach
Not all network paths are created equal. More and more research shows that the shorter paths in the network are more important. Noah Friedkin, Ron Burt and other researchers have shown that networks have horizons over which we cannot see, nor influence. They propose that the key paths in networks are 1 and 2 steps and on rare occasions, three steps. The "small world" in which we live is not one of "six degrees of separation" but of direct and indirect connections < 3 steps away. Therefore, it is important to know: who is in your network neighborhood? Who are you aware of, and who can you reach?
In the network above, who is the only person that can reach everyone else in two steps or less?
Boundary Spanners
Nodes that connect their group to others usually end up with high network metrics. Boundary spanners such as Fernando, Garth, and Heather are more central in the overall network than their immediate neighbors whose connections are only local, within their immediate cluster. You can be a boundary spanner via your bridging connections to other clusters or via your concurrent membership in overlappping groups.
Boundary spanners are well-positioned to be innovators, since they have access to ideas and information flowing in other clusters. They are in a position to combine different ideas and knowledge, found in various places, into new products and services.
Peripheral Players
Most people would view the nodes on the periphery of a network as not being very important. In fact, Ike and Jane receive very low centrality scores for this network. Since individuals' networks overlap, peripheral nodes are connected to networks that are not currently mapped. Ike and Jane may be contractors or vendors that have their own network outside of the company -- making them very important resources for fresh information not available inside the company!
Recent applications of SNA...
Reveal how hospital-acquired infection[HAI] spread amongst patients and medical staff
Map and weave nationwide volunteer network
Improve the innovation of a group of scientists and researchers in a worldwide organization
Find emergent leaders in fast growing company
Improve leadership and team chemistry for sports franchises
Build a grass roots political campaign
Determine influential journalists and analysts in the IT industry
Unmask the spread of HIV in a prison system
Map executive's personal network based on email flows
Discover the network of Innovators in a regional economy
Analyze book selling patterns to position a new book
Map a group of entrepreneurs in a specific marketspace
Find an organization's go-to people in various knowledge domains
Map interactions amongst blogs on various topics
Reveal key players in an investigative news story
Map national network of professionals involved in a change effort
Improve the functioning of various project teams
Map communities of expertise in various medical fields
Help large organization locate employees in new buildings
Examine a network of farm animals to analyze how disease spreads from one cow to another
Map network of Jazz musicians based on musical styles and CD sales
Discover emergent communities of interest amongst faculty at various universities
Reveal cross-border knowledge flows based on research publications
Expose business ties & financial flows to investigate possible criminal behavior
Uncover network of characters in a fictional work
Analyze managers' networks for succession planning
Discern useful patterns in clickstreams on the WWW
Locate technical experts and the paths to access them in engineering organization
Social Network Analysis
社会网络分析 (Social Network Analysis ; SNA)简而言之,你认识的人对你将要认识的人会有重大的影响。尽管我们可以设计程序以强化组织的学习、知识的传播或创新,但是常常难以理解这些方案所达到的效果。我们已经发现运用社会网络分析 (Social Network Analysis ; SNA)--可用于反映人与人之间或部门间重要知识关系,因此特别有助于提高组织中的协作、知识创新和知识传播。
在管理方面,社会网络纪律的成长得益于商业界的三个重要发展:首先,是发现组织内部非正式结构的重要性,它和组织中的正式结构共存。第二个发现是,近年,来网络社群的发展,颠覆了旧有官僚体系及复杂的知识传递,组织变得更扁平;更有弹性;更可以随需应变。第三个是跨组织合作的快速增长,如合资、联盟、多方合作项目等等。虚拟组织产生了一个新的管理问题-就是在没有严格的上下级关系时该如何管理项目。
在这种情况下,社会网络分析展示了可观的前景,有助于组织处理许多典型的情况,包括:
领导选择--谁在人群中是能被信任和受人尊敬的?
任务团队选择 --我们如何将整个组织内有联系的人组成一个团队 ?
合并和收购--不只是两种文化的合并,而是两个独立网络的合并 。
社会网络分析 (Social Network Analysis ; SNA) 和知识管理社会网络分析(Social Network Analysis ; SNA)使得管理者可以想象并理解,一些可能推动或阻滞知识创新和传播的相互关系。信息在一个组织内部如何流动?人们会向谁求助? 有没有出现合并后的下级组织不能有效共享信息的情况?当分析社会网络(个体和连接团体的社会关系)经常会问到这些问题。这些问题的主要特征在于显示社群关系的模式和个体(或组织)之间的相对位置,也因此可以加强知识管理。
知他人之所知在决定是否向某个人咨询信息或意见时,这个人必须对别人的知识、技巧和能力与当前问题的相关性有一定的理解。
尽管由于种种原因,这种理解有可能是错误或有偏差的,但这仍然是决定向谁就某一问题咨询信息或建议的基础。这样,理解一个小组中成员对彼此的知识、技能和能力的了解程度是理解他们在知识共享和创新方面有效的第一步。
能够及时地知道"谁"有哪方面的知识。并可以要求及时地获取"那人"的知识。
透过认知创造可行的知识 当然,单纯的了解并不能帮助知识的传播和创新。人们在传播和创新知识方面有别于传送文件或数据库的方法,就是积极地帮助别人思考他们试图解决的问题。对于那些咨询他人的人来说,有些人愿意先理解别人的问题,然后积极地将他们的知识略加修改以直接应用于问题本身,这样便有助于知识创新。这和一些只是提供简单的信息,而没有积极地解决问题的人形成了鲜明的对比。正如一位经理人所说,"我周围有不少这样的人,他们只是很快地给你一个说法,因为他们自以为很聪明,并且给你一些提示就使你很快地佩服他们,然后他们就可以逃脱解决问题的困难工作。Mike的责任感和思想觉悟并非如此,因为他会帮助你思考问题。"这样,网络的第三个重要因素就是评估哪些人会积极地和别人交流,帮助别人解决问题。
在一个安全的环境中学习 最终,关系具有一些属性,这些属性可以影响交互中出现的学习和创造性的程度。当一个人向另一个人咨询信息时,他们自然地变得易受攻击,因为"寻求帮助暗示着无能力和依赖。"咨询他人,是给一个您所"信任"的人一个权力。所以当一个人对另一个人的信任使得他承认自己的知识缺乏是不容易的,个人和团体都是如此。进一步说,以安全或信任程度为基础,也为相互的探讨和创新提供了空间。以安全或可靠为特征的关系透过创新和学习的空间提高了知识创新的能力。
Gibbs sampling (I)
It is used to generate a sequence of samples from the joint probability distribution of two or more random variables. The purpose of such a sequence is to approximate the joint distribution or to compute an integral. The algorithm is named after the physicist J.W.Gibbs, in reference to an analogy between the sampling algorithm and statistical physics. The algorithm was devised by Geman and Geman, some eight decades after the passing of Gibbs, and is also called the Gibbs sampler.
Gibbs sampling is applicable when the joint distribution is not known explicitly, but the conditional distribution of each variable is known. The Gibbs sampling algorithm is to generate an instance from the distribution of each variable in turn, conditional on the current values of the other variables. It can be shown that the sequence of samples comprises a Markov chain, and the stationary distribution of that Markov chain is just the sought-after joint distribution.
Gibbs sampling is particularly well-adapted to sampling the posterior distribution of a Bayesian network, since Bayesian networks are typically specified as a collection of conditional distributions. BUGS is a program for carrying out Gibbs sampling on Bayesian networks.
Metropolis-Hastings Algorithms (I)
The M-H algorithms can draw samples from any probability distribution p(x), requiring only that the density can be calculated at x. The algorithm generates a Markov chain in which each state x(t) depends only on the previous state x(t-1). The algorithm uses a proposal density Q(x', x(t)), which depends on the current state x(t), to generate a new proposed sample x(t). This proposal is 'accepted' as the next value (x(t+1)=x') if u drawn from U(0,1) is
The original Metropolis algorithm calls for the proposal density to be symmetric (Q(x;y)=Q(y;x)); generalization by Hastings lifts this restriction. It is allowed for Q(x', x(t)) not to depend on x' at all, in which case the algorithm is called "Independence Chain M-H" (as opposed to "Random Walk M-H"). Independence chain M-H algorithm with suitable proposal density function can offer higher accuracy than random walk version, but it requires some a priori knowledge of the distribution.
Now, we draw a new proposal state x' with probability Q(x'; x(t)) and then calculate a value
a = a1a2
wherea1 = P(x')/P(x(t))
is the likelihood ratio between the proposed sample x' and the previous sample x(t), and
a2 = Q(x(t); x')/Q(x'; x(t))
is the ratio of the proposal density in two directions (from x(t) to x' and vice versa). This is equal to 1 if the proposal density is symmetric. Then the new state x(t+1) is chosen with the rule
